Building Products in the Age of AI

.webp)
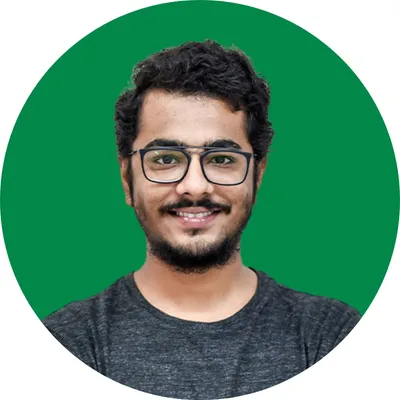

Introduction
In this conversation, Yash Shah and his co-founders discuss the challenges and strategies of building SaaS products in the age of AI. They explore how to identify AI potential within existing products, the importance of understanding customer pain points, and the decision-making process between integrating existing AI models versus creating custom solutions. The discussion also covers the significance of building a strong AI talent pool, real-world examples of successful AI implementations, and pricing strategies for AI features. Finally, they address the future of AI in the SaaS industry, debating whether the current hype represents a bubble or a sustainable trend.
Key Takeaways
- AI is transforming the SaaS landscape, akin to electricity.
- Understanding customer pain points is crucial for AI integration.
- Start with existing AI models before considering custom solutions.
- AI should enhance existing use cases, not create new ones.
- Building a strong AI talent pool is essential for success.
- Real-world examples show effective AI use in SaaS products.
- Pricing strategies for AI features should reflect their value.
- The market's acceptance of AI features is critical for revenue.
- AI will become a standard expectation in software products.
- The future of AI is promising but will stabilize over time.
Transcript
Yash Shah (00:00.515)
Yeah.
Yash Shah (00:04.108)
in real life? Yeah.
see. So we should start.
I'm just making sure that I don't get a call or anything like that while this is going on. It's the Apple connected ecosystem. When your phone rings, it starts to ring everywhere. Your watch also vibrate, your laptop also vibrate, like everything. It just makes that. Yeah, just like Eaves, right? It rings. There's no way that you can, there's no excuse. There's no way to miss. But okay, so we're here.
And let's start. So hello and welcome to Momentum of SRs. My name is Yash, and I'm joined by my co -founders Harsh, Jay, and Kaushik to discuss topic of the week, which is building SaaS in the age of AI. Our goal is to provide you with actionable insights and practical strategies that you can apply to your own products. Throughout the session, we encourage you to engage with us by asking questions and sharing your thoughts. This is a fantastic opportunity to learn from each other and give you insights that can help drive your SaaS initiatives forward. So let's get started.
Jay, Harsh, Kaushik, how are we doing today? Nice. Good, good. Yes, good. Quite good. But it's been a hectic day to push on. It's been a hectic day. Yeah. Perfect. Perfect. So let's talk about it, building SaaS products in the age of AI. What do you guys think? I mean, we are in the heat of the moment, right?
Yash Shah (01:40.18)
everyone is in this, if you don't build you are in a bigger, bigger FOMO and bigger you feel left out. That's the situation for people who are building, people who are investing, everywhere it's happening. It's almost like it's the new electricity. yeah, it is. And so I was having a conversation with someone a while back and one of the things that they said very interestingly was
was that a lot of enterprises are doing AI POCs and building MVP using AI and they have been given budgets by the higher -ups saying, hey, here's half a million dollars, make something useful using AI and things like that. But one of the trends that we're also seeing is that these enterprises are finding a hard time taking those POCs and MVPs into production across the whole
organization. And we do know what is the core reason as to why that is happening, but that an interesting trend. And so I thought I will mention that and probably if and when we talk about it, we would love to explore a little on that trend as well. But yes, like I think to kick off one basic question that I always had with respect to since this, you know, the whole
AI thing started is that how, a SaaS product owner or as a SaaS product, who runs a SaaS product, so how do I identify the potential of AI within the scope of my own product that's there? how do I even start with that is the question. So that's a great question and so a good way to go about it is,
The best way to go about it is to treat AI as a piece of technology. So from that standpoint, technology is something that is a way to fulfill an existing use case. So talking to your customers, going back to the basics, talking to your customers, first principles, understanding what are their pain points. So like, what are the jobs to be done? What are the pain points in accomplishing?
Yash Shah (04:05.474)
those jobs, what do they stand to gain if those jobs are accomplished well. And then just essentially you would have already done this exercise if you're running a successful SaaS company. Some of those JTBD or some of those pains and some of those gains, you were not able to incorporate into your product because the technology didn't exist at that point of time. And now in some way, shape or form it exists. And so you can always go back and say, okay, hey, here are some
use cases, certain journeys that our customers actually want to perform using our product. We were not able to fulfill that because technology didn't exist. Now it does. And so how can I bring that in? The biggest mistake that a lot of SaaS founders I see making is that they look at AI and then they classify as to what are the things that AI can do. And then they try and bring those technology pieces within their product, hoping that
that customers will just start using it. And that's the exact opposite approach of how it should. So it should always be customers, main points, technology, solution. It should never be technology, solution, and then let's look for the problem that this solves. So that's the best thing. The other comment that I'd also make over here is, it's always a little, I find it a little amusing because
every step that the technology makes, like every jump that the technology makes, you as a founder, who's building a company is forced to take a step back, and then go back to the first principles and then sort of kick up from that. So that's a little interesting, find that piece a little amusing. that's, if you're an existing SaaS company, that's what I would recommend. And I would also give a couple of examples. I think Notion
ClickUp, even in certain cases, Google Drive, they have done a phenomenal job of doing the same thing. I think Microsoft is getting there, fingers crossed, hopefully. They have a very large suite of products. But yeah, so Notion ClickUp, like they have done an amazing job of looking at the pain points and then figuring out the technology to build it within their own products.
Yash Shah (06:34.818)
Yeah, so yes, so in this process, so there are two ways, right? Such like there are AI reports are available, right? Such like the OpenAPI, OpenChainGPT, like a LAMBATO, right? Otherwise, first way is we can integrate these models, right? Otherwise, we can create our own custom model for our own use cases, right? So what do you think, what initial stage, right? Where we just have...
run this or we are just want to start the business right or we have some amount of revenue right. So, in which stage we have decide we have to decide whether we have to integrate available model or we have to create our custom model for our use case. So, if you are starting today I would argue that that the second option does not exist right. So, unless you are Elon Musk or you are Jeff Bezos.
like those people start or Mark Zuckerberg, right? So those people starting today, the second option exists. But if you're just another entrepreneur who did a couple of million dollars in your previous company, or who's moving out of a job and starting to a SaaS product because you identify an opportunity, the second option doesn't exist, right? So the goal is to use existing LLMs and then try and generate an output that is meaningful for your customers.
and is well integrated into the workflow. And then over a period of time, you will start to see some amount of feedback, some amount of expectations that your customers have. And it's a gradual approach. So if you integrate with an existing LLM today, you started with the first option. And then when you start to realize that this piece of integration in and of itself at the unit level,
is offering a positive ROI to the customer. That is when you start to customize or verticalize fine -tuning, fine -tune the LLM. So that's how you should go about it. The other piece that I'll also mention over here is that don't attribute the non -AI revenue to the AI part of your product. So when you're making a decision of
Yash Shah (08:59.406)
fine tuning and making the decision of investing into doing that exercise, only attribute the revenue that you are being able to generate from the customer for that specific piece. So that's, so like if you're doing $100 ,000 in MRR without all the AI and with AI, if you start to do $110 ,000, the AI piece is only giving you $10 ,000. So look at your account expansions that are happening because of AI. Look at your
So look at the additional revenue that is coming in through AI and then only allocate that piece towards this. Because of a trend of a lot of companies wanting to or starting to build in AI, specifically for an existing company, I see a lot of founders who started to build it, who started to deploy capital on AI related initiatives. And then now there's no way to monetize it.
But now what has happened is they've already set the expectations. It's another custom is already used to the AI piece, but is not willing to pay for it. If you remove the AI piece, they'll give you a negative review. But you don't have the ability to improvise it as well. So please don't let that happen. So like I have also been reading a lot of course related to, you know, moving to that particular point to mention that a lot of
I mean, there has been a lot of funding happening for SaaS companies that are having AI as the selling point. But then still, has been no ROI so far, much, as per the expectations from investors' standpoint. So that, again, tells the story of the challenges that are being faced. I had one simple question from my side with respect to, because of AI, there are a lot of tools, because of which, especially for early -stage SaaS.
MVP development or GSI development has become much faster. So for early stage, it could be advantageous when it comes to companies which are already into SaaS, but now they are planning to build a counter product to it just to have more user acquisition. What should be the focus? they also opt for building using AI, or they should just simply go with the traditional way and have a tech stack which is strong already and we just proven.
Yash Shah (11:24.864)
results before. So not building with AI, I'm afraid is not an option. So while I agree with what you are saying in terms of making sure that the AI use case is economically viable, a lot of companies have not been able to do that. But one area or one sector where it has been tremendously successful, I'd argue is the software engineering piece.
So the co -pilots of the world and the cursors of the world have done a brilliant job of making sure that AI use cases are implemented in the workflow well, and they actually generate a positive ROI. And that customers, which is the software engineering companies or companies that are building products, they are willing to pay for it. So if you're building a software using AI,
as a part of the software development processes, it's not a choice anymore. It's something that you have to do because it's proven to, it's absolutely proven to sort of generate better outputs, to generate better quality of code, to make sure that there are lesser bugs and things like that. And actually last week for all the people who are viewing and listening, last week, Harsh was talking about
How can a SaaS company actually use AI as a part of product development to accelerate their product development? And so you can always head out to our website, click on Resources, Office Hours. You'll be able to find Harsh's session there. And if you want to do a deep dive on that front, we already have that done for about 30 or minutes in the page.
Yash Shah (13:14.988)
Yeah. So I have a little other side of this question. I mean, of this topic. In respect of you are a product company or a service company, how do you build a strong AI talent pool within your organization? Such that there are people to build and maintain this thing. So because I keep hearing this very often that skilled professional in this field is
very tough to get them as well as to also to maintain them within the organization and also to keep them continuously in run with the trend and everything. So how do how do you do that? So that is a great question and I would not claim to be an expert on this topic because while I am figuring out
Everyone is figuring out right so everyone in the world is figuring out because this whole thing is only about a year and a half old and and so So so how do you retain the AI talent or how do you develop AI talent? Is something that a lot of executives and organizations are trying to find an answer here are a couple of ways to to To make sure that your talent is is working on AI or like how do you choose the people?
who will work on this particular initiative. There are a couple of qualities that I'd look for. So I'd look for people who are curious. I'd look for people for whom at this point of time, they're early -ish in their journey. so there's very fine balance where some amount of experience means that they are mature. And however, they are a little early so that they are not ruined by experience also.
So learning is a priority, but then at the same time, they understand the requirement of building a process or a system that is maintainable and that is sustainable as well. So look for generally curious, a little bit of experience with people for whom learning is a priority. That's the first thing that I would say. From an attracting talent pool, I think the fundamentals stay the same.
Yash Shah (15:38.772)
if you as an organization are able to make a dent in a particular industry, if you're able to showcase to a team member that this is the impact that their work will be able to generate on the industry in general, that is known to motivate most individuals. In terms of retention of the talent, I believe that as an industry in and of itself, we've not reached to a point where it
it becomes a question because it is just so early that people leaving, like people who acquired skills in AI moving to other organizations has not become sort of a reality at this point of time is what I would say. The other counterpoint that I would also make is that
Retaining and acquiring talented folks who are able to understand and make sure, the eye for detail for the use cases that AI can solve. So, for the people who are able to, so I'll give you an example. The people that you look for are people who have great judgment. And the way to define judgment is ability to make the right decision.
with as little amount of variables as possible and then to be proven correct in hindsight. So if the wisdom of hindsight agrees with the decision that you made in the past, then you are a person with great judgment. And having those people who have great judgment, where they have eye for the detail and great judgment as well, so that they are able to sort of declutter
everything that is happening in the space, focus on use cases that need to be solved and then map them with the capability that AI as a technology has. Retaining, developing, acquiring that talent pool, I would argue is more difficult and that's something that an organization should focus on.
Yash Shah (17:54.04)
Got it. So, yeah, so yes, think previously you talked right.
in any SaaS product, instead of finding the AI use case, first they have to find the use case and then after how can implement AI in that particular use case. So can you give us some, we can say the two three SaaS product where they have good use case and then after they implement AI in the use case, they can implement the AI in their particular use case. Just two three samples for that.
Yeah, absolutely. So I'll take an example of a founder called Sebastian, who ran a SaaS company called Alfana. He was on our podcast called Breeding Momentum. You can check it out on YouTube. Just search for Breeding Momentum, and you'll see our podcast as well. So as an example, in absence of Alfana, what used to happen was that we'll create this video. We'll create this asset.
And then there will be a person who will be required to write subtitles. There will be a person who will be required to edit the video. There will be a person who will be required to create transcripts. There will be like the same person, but that person is also writing the blog post. That person is converting it into social media carousels. That person is figuring out how can this be sent out as an email newsletter or whatever the case may be. So the conventional wisdom in the content creation industry is that once you have a pillar of content,
You want to have a team that is going to repurpose the content into different formats for Instagram and LinkedIn and email and blogs and all of those things. So that was already happening in the organization. So that's one part of it. The second part of it is that we know that AI can listen, watch, read and understand. So we know that AI has the capability to do these things.
Yash Shah (19:57.486)
So what Stephen, there are a couple of other companies apart from Alfana who are also doing this, but I'll give a shout out to Alfana because he was on our podcast. So one of the things that Alfana does is that you record the episode, upload it into Alfana and it will create 30 different pieces of content. And so that's a real life example. And this should not come as a surprise. Stephen used to run a digital marketing agency. He's not a tech person.
He doesn't write code, he doesn't do any of that. He used to run a digital marketing agency. He had people who were doing this for his clients and then wanted to optimize for it. And then he put two and two together. So good judgment, good eye for detail, put both of those things together and then built out alpha. So that's one example. And there are a lot of other examples as well. So I'll give you another example. So as an example,
One of the challenges that we have at Momentum as well is that we have a lot more people who are applying for opportunities than we have open positions. So on our website, we used to have careers page highlighted. Now we don't because we are inundated with the people who are looking to work with us, who looking to apply, and we don't have enough positions even though we are trying to grow as quickly as we can. And so the challenge that you yourself,
had or Koshik had when Koshik was looking for UI, UX, or Hush, he was looking for engineering, was that here is the job description that I have. And then I have so many applications to look through. I have 25, 30, 40, 50 applications to look through for a particular job profile. And so there's a platform, the own HR system that we have, essentially looked at this as a problem for a lot of other organizations as well.
And then build a small piece which is where all the resumes are also passed through AI and all the job descriptions, obviously they are written on the platform so they already know what those job descriptions are. And the only thing that they do is they just sort the resumes in order of the match that their resume and the job description make. And so this is such a small thing which is where why are all resumes equal?
Yash Shah (22:19.746)
They shouldn't be equal. You should be able to sort them in the priority with how much percentage of overlap do they have with the requirement that you have. So this is another example. And so there are n number of examples, which is where if you have very tight understanding of the use case, if you good judgment, you'll be able to put two and two together and then build out solutions that are actually meaningful and helpful.
Interesting. So going to the earlier discussion that we had, like you mentioned that there are a lot of people when SaaS companies are starting to charge for AI use case, they are not ready to pay and they have the negative reviews and everything. So is that a hint that let's say you are enabling AI component, you consider it in at least your second and third year of pricing itself and you do not
keep it as an add -on or something. So what I'm trying to look for is, is there a hint for any SaaS founders when they are just implementing any AI component in their existing SaaS product, what they should keep in mind while making their pricing or while updating their pricing? So I'll tell you what. So it's extremely arrogant for a founder to think that the market is wrong.
The market wants what it wants and your job is to build for what the market wants. The reason why the customers are not paying for something that you have built is not because the customer doesn't want to pay. The reason why the customer is not paying is because they don't want what you've built. And that may be a very harsh reality a lot of founders may not be able to accept and understand and live by. So, because a lot of people are paying for
for AI. A lot of people are paying for the use cases that AI solves for. There are a couple of things that may have gone wrong. So the first is you may not have understood what is the use case that this AI should solve. Second could be that you may not have priced it properly. There's no relationship with the ROI that this particular piece of AI that you incorporated into your product has. There's no relationship that's established over there. There are n number of problems that could exist.
Yash Shah (24:40.59)
And so, it's not like the customers don't want to pay for it. It's just that the founder of the SaaS company has not identified the right use case where a customer will actually have like need the need that piece so much that they'll pay for it. So, yeah, so that's sort of what I would say. From a pricing strategy standpoint, think AI at this point of time is we are so early in the journey that it is still cost based pricing.
And so what we have seen organizations do often is that whatever it is costing them, they are marking that up by 30, 40 percent and then offering that to the customer. And this is true for any industry in its nascent and early stages, that they are always cost -based. And so I would recommend that from a pricing strategy standpoint, you may not want to go against
your competitors or you may not want to go against the market expectations and do value benchmarking and value -based pricing. You just want to do what other people are doing. Spend all of your innovation budgets on AI. Don't innovate on the pricing strategy. If everyone's doing cost -based pricing for AI, just do cost -based pricing. There will come a time when it turns into value -based pricing and at that point of time, you can always pivot.
Yash Shah (26:11.054)
I have a question. my question is, do you think this is a bubble? Because I know it's a very naive question to ask. know many people have been on the internet asking this. But the numbers sort of say that because we have close to 200. I mean, have read it somewhere that there's close to $200 million pumped in from investors.
in the West into the field to develop upon AI, but how much has been, know, come out of it is significant weakness. So, that means it is a bubble, So, good question here and thank you for the assumption that I am smarter than the people who have pumped in billions of dollars into AI to be able to answer that.
I'm sure there is a compliment in there somewhere. So here's what I would say. So I wouldn't say that it's a bubble. I'll for sure say that it's a balloon that will eventually revert to a size that is smaller than what it appears to be. So that's sort of what I would say. So it's not like Web3 or it's not like
you know, meta or I mean, meta is a company, it's not like, whatever AR VR, where it will actually burst, right? So it will, like there's going to be a lot of excitement and then it will go to levels that is so small, very, very insignificant, right? I don't think that's what's going to happen with AI. I think that the new reality is going to be that softwares in an of
themselves will come with AI related things and it will become a zero experience sort of an understanding. So the amount of chatter that we have around AI will die down over the next few years. At the same time, the software's, you will expect just the way that you expect a phone to come with a camera, you will expect the software to come with AI and just the way that the camera improves from one generation to the next and then.
Yash Shah (28:35.148)
the more generations that happen, the smaller are the improvements. I think that's essentially what will happen with AI as well. But it is going to be an important and a key component, just not as big in life ordering as it seems to be. We also have to understand that a large part of the industry is being run by SEO people. And the incentive that
that SEO people are marketeers largely, and me being one of them. The incentive that marketeers and SEO people have is to get eyeballs. And when you have eyeballs and when engagement and attention and eyeballs is a metric that a marketeer is gunning for, they will often make tall claims that are not, that are like disjointed from the reality as well. But that's how the world works.
That's what it is. But I think eventually it will be a huge impact, but it will be smaller than what it feels like today is what I would say. OK, we have time for one last question if there are any questions or else we'll close. I think we have a chat message as well. is someone saying good evening. Hey, good evening. Thank you for joining in. But any questions from any other questions?
If I ask, it may take some longer time and it may be little away from the main topic that we have. we can keep it for the next episode or the next session. it. Awesome. awesome. So great. think this I would I hope that this has been a meaningful conversation for for all of us who are who are here. And I hope I was able to give some amount of answers, maybe not all the answers, but maybe some things that
that might be helpful when you are thinking about building anything with AI. I'd like to thank all the people who joined in for this episode, whether you're on LinkedIn or YouTube, do drop a note in the comments so we know that, you know, what, if there are any changes, if there are any other formats that you'd like to see us do, do let us know in the comments here as well. And just as a way of encouraging us, right, if you can like, share, subscribe, do whatever, right, so.
Yash Shah (30:57.39)
As I shared a little earlier, we are a market here and we're trying to grab your attention and trying to have your eyeballs on this conversation as well. But thank you for joining in and until next time.